Confidence vs. Accuracy in AI: Why Both Matter
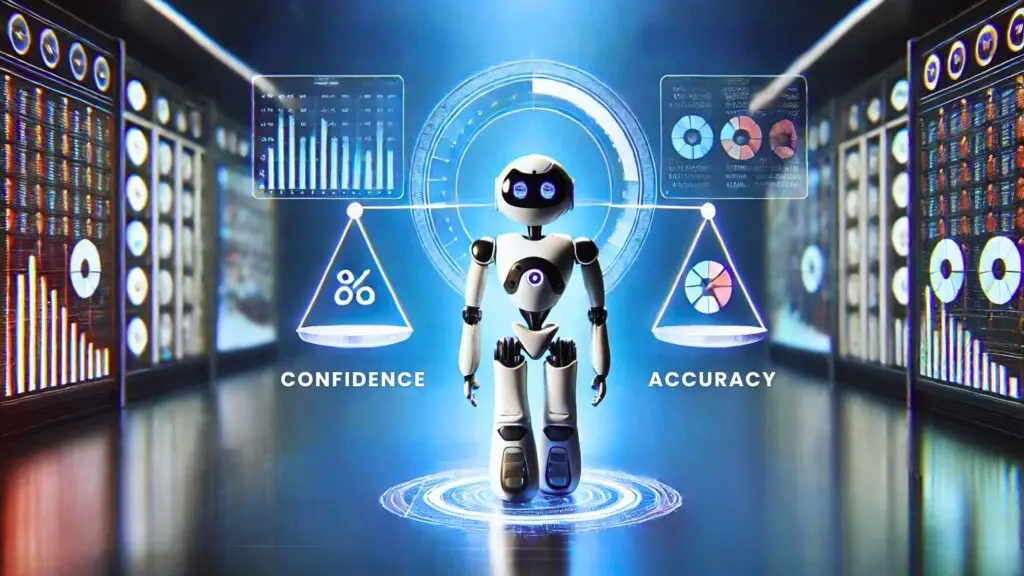
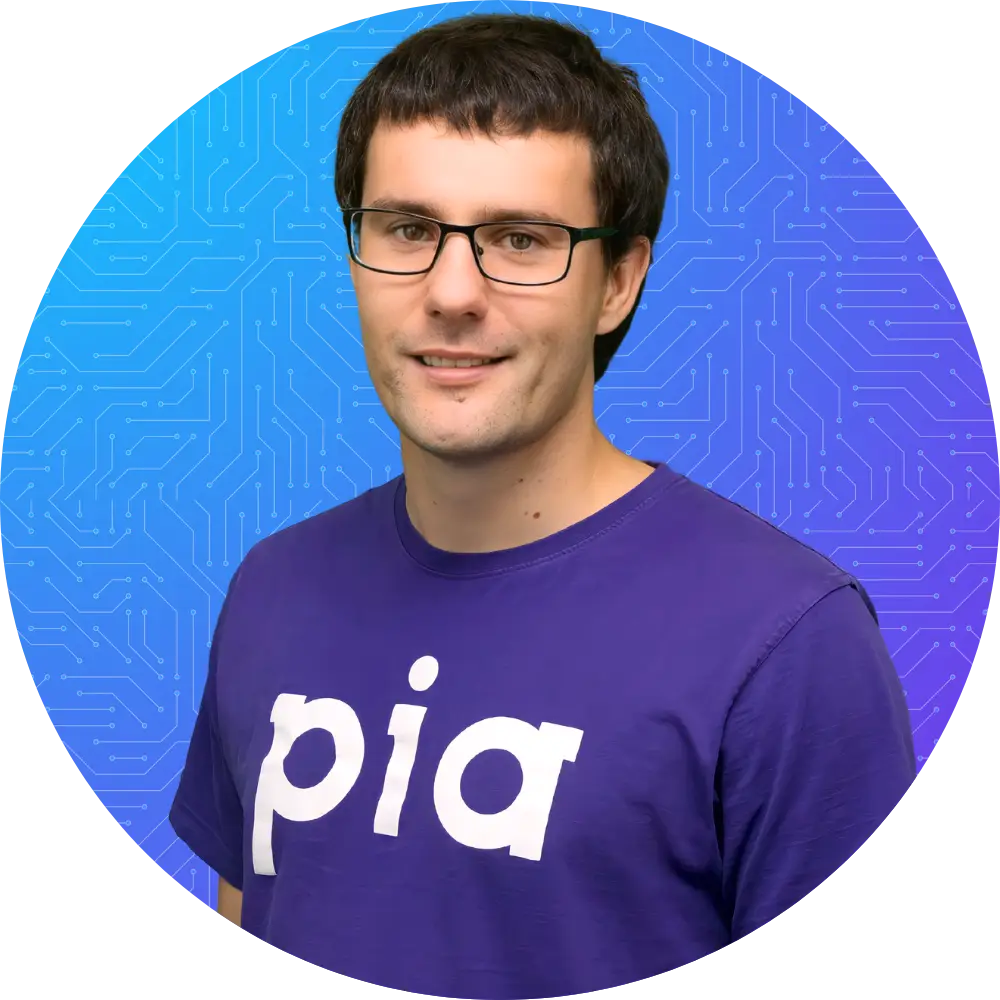
Aron Hardy-Bardsley
Chief Technology Officer
When it comes to effective automation, two crucial concepts often get tangled: confidence and accuracy. While both play a fundamental role in shaping AI-driven automation, understanding the distinction between them is the best path to leveraging AI as an autostart for your ticketing process.
Let’s break it down—what do these terms actually mean, why do they matter, and how can businesses, particularly Managed Service Providers (MSPs), make informed decisions about AI adoption so that they’re better prepared to use these tools to improve their ticket processing and so much more?
Confidence vs. Accuracy
At first glance, confidence and accuracy might seem interchangeable, but they serve very different purposes.
Confidence is how sure the AI model is about its decision. It’s a probability score that indicates how strongly the model believes a particular answer or classification is correct. However, a high confidence level does not guarantee accuracy. AI can be confidently wrong.
Accuracy is the percentage of times the AI gets it right. A model with high accuracy makes correct predictions most of the time, even if its confidence varies.
For instance, an AI model might classify a ticket as a “hardware issue” with 95% confidence, but if the true problem was a software bug, then despite its high confidence, the classification was incorrect. Conversely, an AI might classify an issue as a “network problem” with only 60% confidence, but if it’s actually correct, then its accuracy is still intact.
3 Ways to Maintain Balance
When AI is used in critical processes like triaging IT support tickets or automating responses, it is essential to strike the right balance between confidence and accuracy.
- Avoid confidently wrong decisions: A key risk with AI automation is that when models make incorrect decisions with high confidence, those errors can be amplified across a system, causing inefficiencies or even harm. For MSPs, misclassifying IT support tickets at scale can result in delays, misrouted requests, and customer dissatisfaction.
- Build trust in AI: If AI makes too many confident mistakes, users will quickly lose trust in it. This is why transparency and control mechanisms are critical—users need visibility into how AI makes decisions and the ability to adjust its parameters.
- Balance risk and automation: Businesses have different risk tolerances. Some may prefer AI to act conservatively, only making automated decisions when it has very high confidence. Others may accept a slightly lower confidence threshold to speed up operations, relying on human oversight when needed.
Finding Confidence in AI
For MSPs and other businesses adopting AI, the key to trust and effectiveness lies in understanding and controlling confidence thresholds. Here’s how:
- Look for AI models that provide both confidence and accuracy scores. A high-confidence result is only meaningful if it comes from a model with a strong track record of accuracy.
- Choose AI solutions with transparency and control. AI should provide clear insights into why it made a decision and allow users to adjust settings based on their risk tolerance.
- Start small and scale up. Instead of turning on full automation immediately, businesses can begin with controlled trials, gradually increasing reliance on AI as trust in its accuracy grows.
Final Thoughts
Confidence and accuracy are both essential in AI, but they are not the same. A truly effective AI system balances confidence with real-world accuracy while giving users control over how and when decisions are made. For MSPs and IT service desks, this balance can mean the difference between a smoothly automated workflow and a costly operational headache.
By carefully evaluating AI models and their decision-making processes, businesses can harness AI’s potential without losing control over critical operations.
More to explore
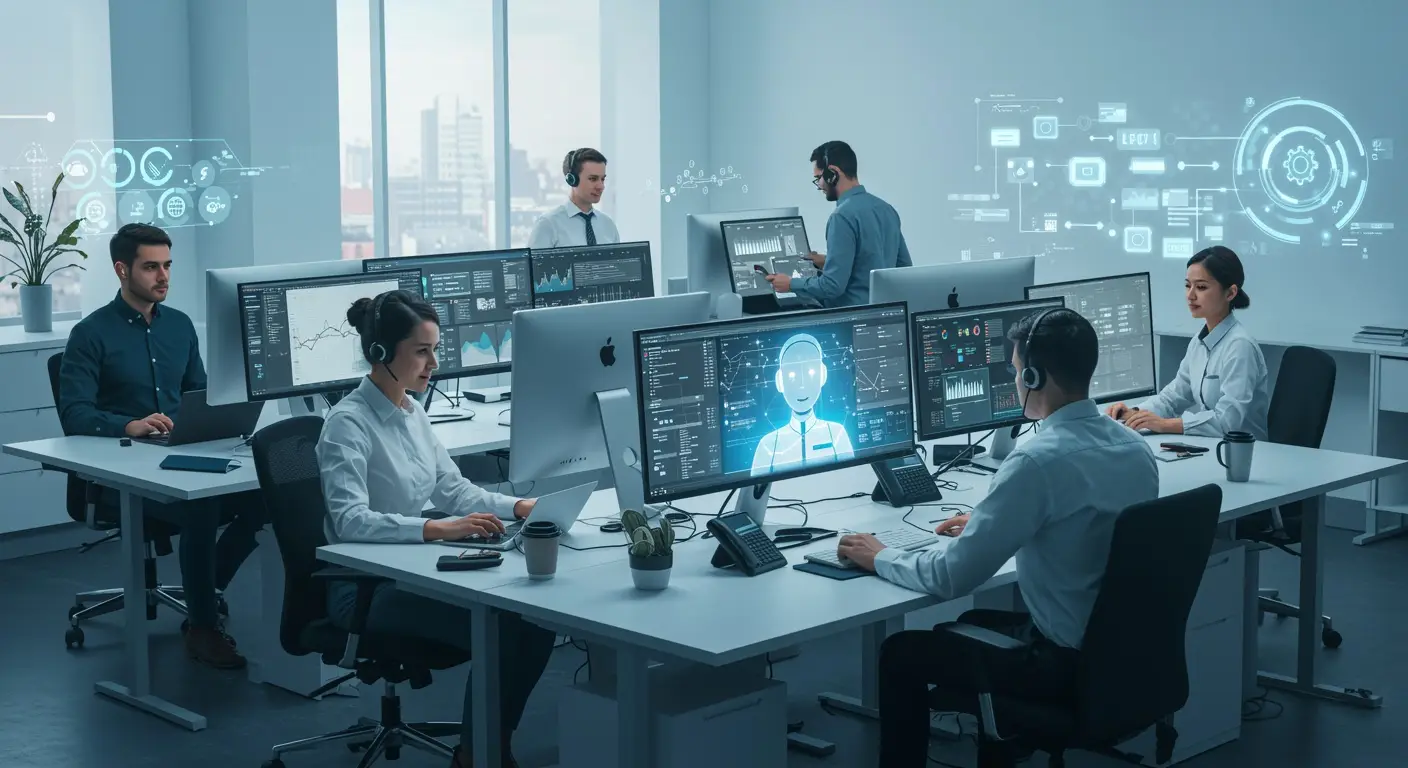
How to Use AI & Automation in Your IT Help Desk
AI is revolutionizing IT help desks by automating repetitive tasks, accelerating ticket resolutions, and helping MSPs scale operations without increasing headcount.
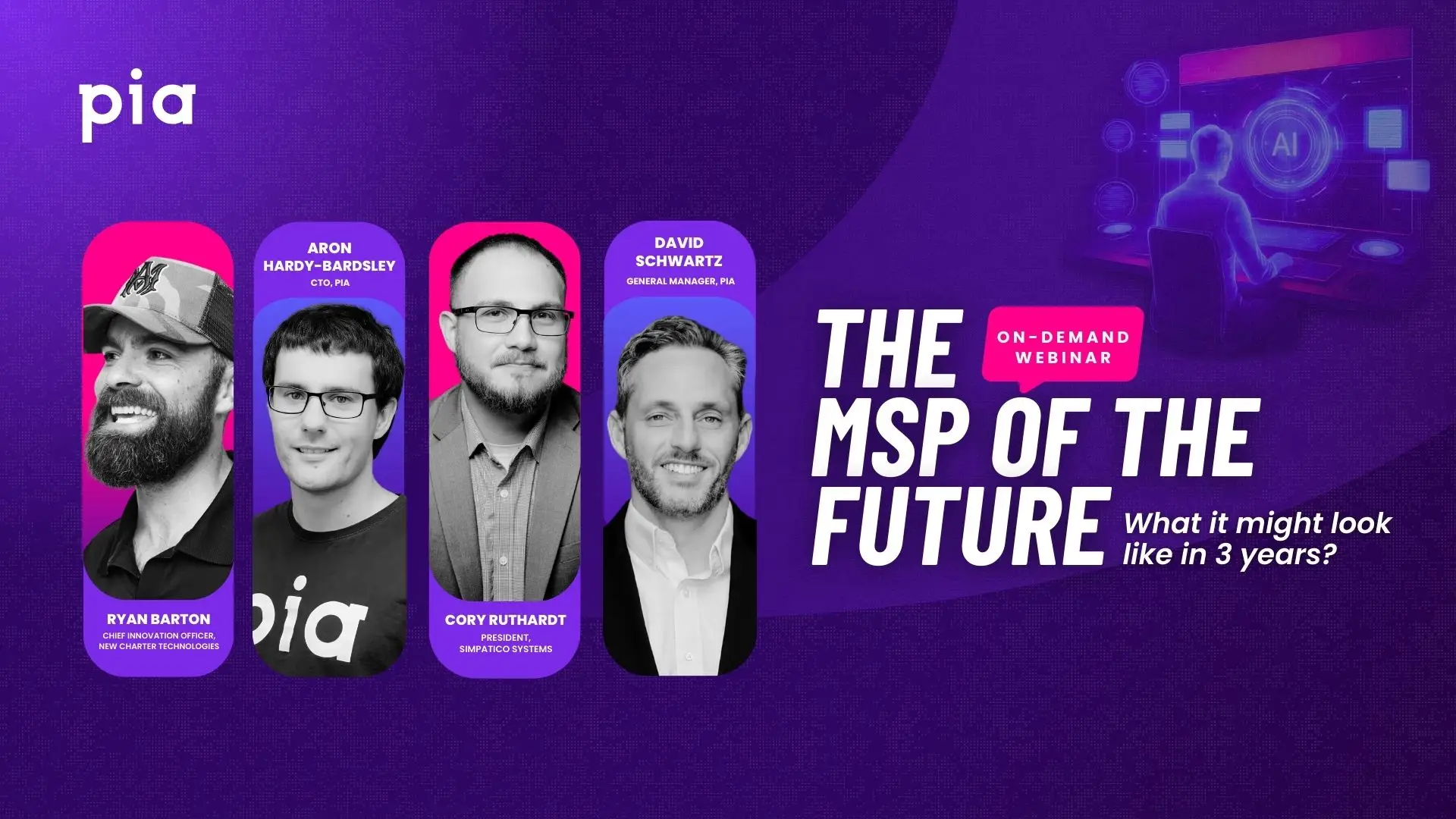
The MSP of the Future: What Might It Look Like in 3 Years? Webinar | On-Demand Webinar
Discover how AI is shaping the future of MSPs in the first episode of Let’s Talk AI for MSPs: A Pia Series with insights from
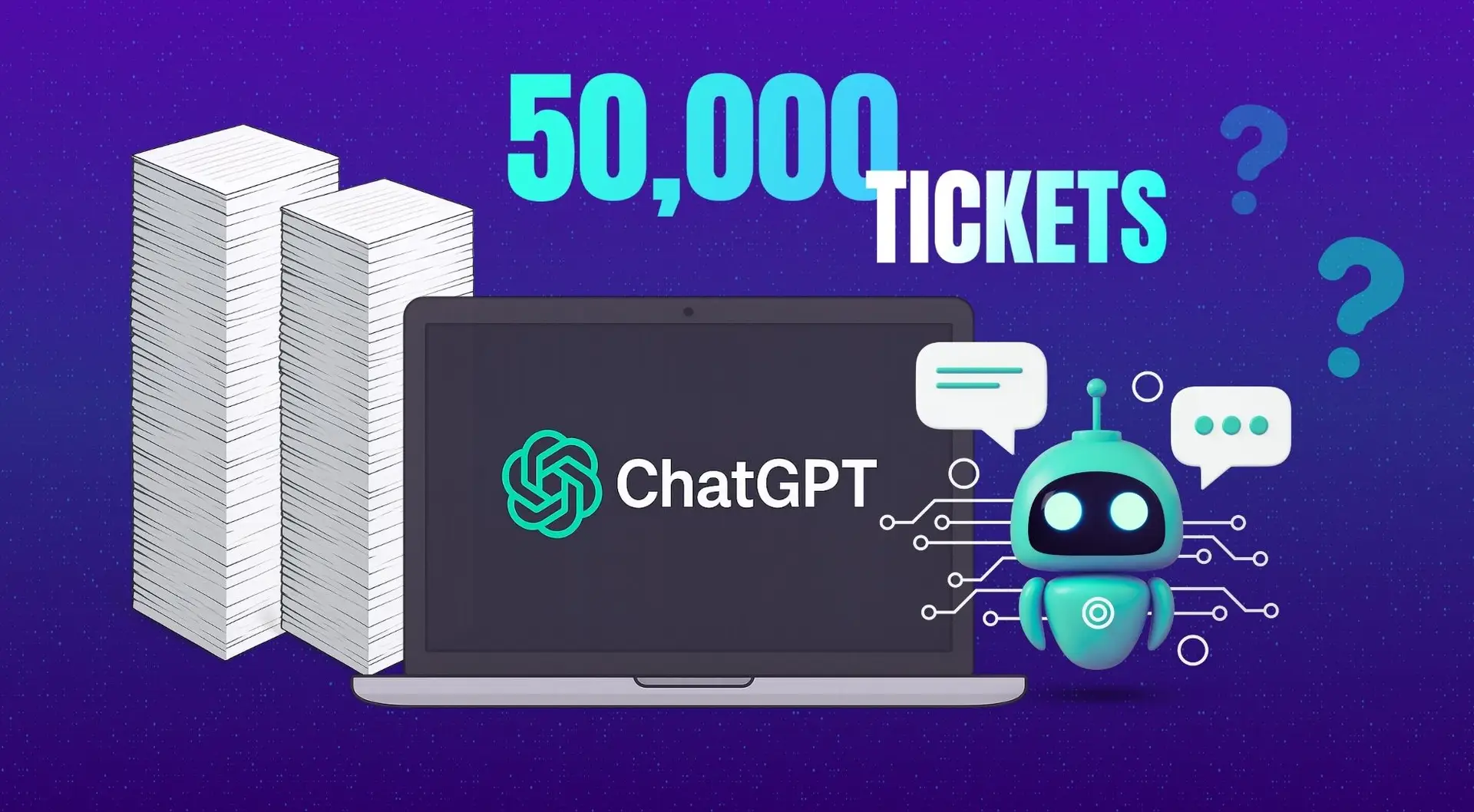
We Gave ChatGPT 50,000 Tickets – Here’s What Happened
We fed 50,000 real service tickets into ChatGPT to test its classification accuracy. Here’s what worked, what didn’t, and what MSPs should know.